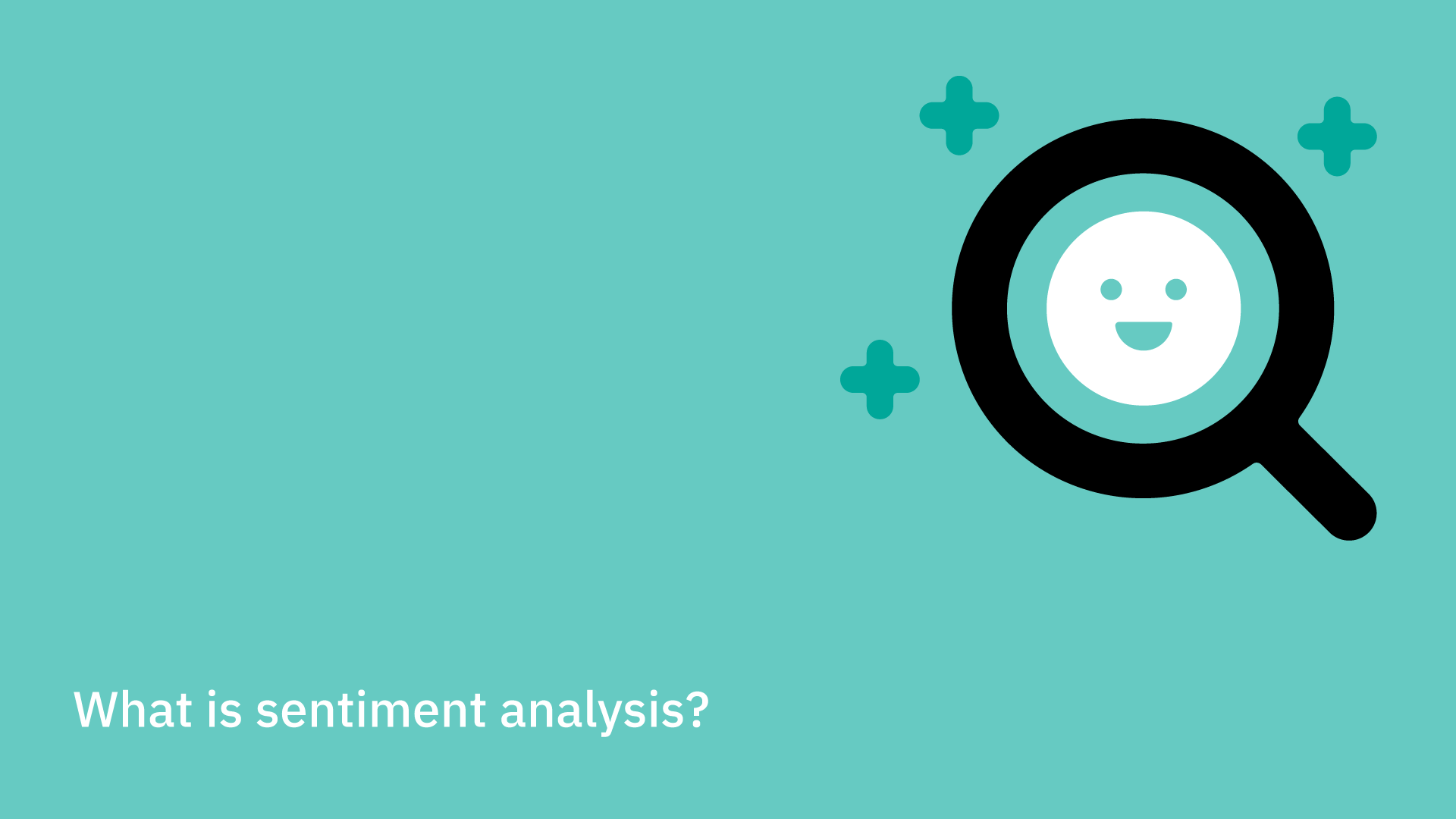
What is sentiment analysis?
Updated on January 15, 2025
Imagine this. You’ve just hung up the phone on a customer service representative who didn’t do the best job in helping you solve your issue. You feel frustrated, and you want someone to know it. But alas, the conversation is over, and to file a complaint you’d have to go through the effort of searching, typing, sending, and so on.
Here’s another example. Remember the infamous Kendall Jenner Pepsi commercial? It ignited a firestorm of negative reactions online, leading to the ad being pulled within 24 hours.
This is a prime example of how sentiment analysis, particularly with the power of AI, can dramatically impact business decisions. It’s a tool that’s been in use in the CX world for many years, but never before has it been as crucial, evolved, and insightful as now.
So, what exactly is this game-changing technology? Let's dive in.
Understanding the basics: what is sentiment analysis?
Sentiment analysis, also known as opinion mining, is the process of using natural language processing (NLP) and artificial intelligence (AI) to determine the emotional tone behind words. It goes beyond simply identifying whether a statement is positive, negative, or neutral; it delves into the subtleties of human expression to understand opinions, attitudes, and feelings within text or speech. According to a 2019 study by CIO, unstructured information accounts for around 80-90% of all digital content.
Think of it as giving machines the ability to "read between the lines" – a crucial skill in today's data-driven world where businesses are bombarded with feedback from customers, employees, and the public. Sentiment analysis helps companies make sense of this vast amount of unstructured data, providing valuable insights that can drive decision-making across various departments.
In the world of customer service, this is an exceptionally useful tool for determining levels of consumer satisfaction, agent effectiveness, and even brand loyalty. It can support in identifying gaps in service or knowledge, streamline operations and processes, and even provide up- or cross-selling opportunities and customer retention activities.
With the advent of sentiment analysis AI tools, the capabilities of registering, reading, and reacting to customer behavior have exploded into a whole new era.
What is a sentiment analysis tool?
A sentiment analysis tool is a software application that leverages the power of artificial intelligence (AI) to automatically analyze text or speech data and extract insights about the underlying emotions and opinions. It's like having a digital assistant that can read through thousands of customer reviews, social media posts, or survey responses and tell you whether people are generally happy, frustrated, or neutral about a particular topic.
AI plays a pivotal role in sentiment analysis tools, particularly in the areas of natural language processing (NLP) and machine learning. NLP enables the tool to understand the nuances of human language, including slang, idioms, and sarcasm, while machine learning algorithms allow it to learn from large datasets and improve its accuracy over time. With AI, sentiment analysis tools can process vast amounts of data quickly and efficiently, providing businesses with valuable insights in real time.
The impact of AI on sentiment analysis tools is transformative. It has made sentiment analysis more accessible, accurate, and scalable than ever before. Previously, sentiment analysis was a manual and time-consuming process that required human experts to read and interpret data. With AI, sentiment analysis tools can automate this process, freeing up human resources for more strategic tasks. Additionally, AI-powered tools can analyze data in multiple languages, making it easier for businesses to understand global customer sentiment.
By leveraging the power of AI, sentiment analysis tools are revolutionizing how businesses understand their customers, track brand reputation, and make data-driven decisions. They provide a valuable window into the hearts and minds of consumers, allowing businesses to respond to feedback, improve their products and services, and build stronger relationships with their customers.
The inner workings of sentiment analysis (with AI).
Text sentiment analysis: deciphering the written word.
Text sentiment analysis has become a cornerstone in understanding and enhancing the customer experience. Companies harness this technology to sift through vast amounts of text data. By gauging the sentiment expressed in these texts, businesses can gain invaluable insights into customer satisfaction, pain points, and preferences.
For instance, a hotel chain might employ text sentiment analysis to monitor online reviews and social media chatter about their properties. By identifying negative sentiment related to specific aspects like cleanliness or staff behavior, they can promptly address those issues to improve customer satisfaction. Similarly, an e-commerce company can analyze product reviews to understand which features are most valued by customers and which ones need improvement. This enables them to tailor their product offerings and marketing strategies to better align with customer expectations, ultimately driving customer loyalty and business growth.
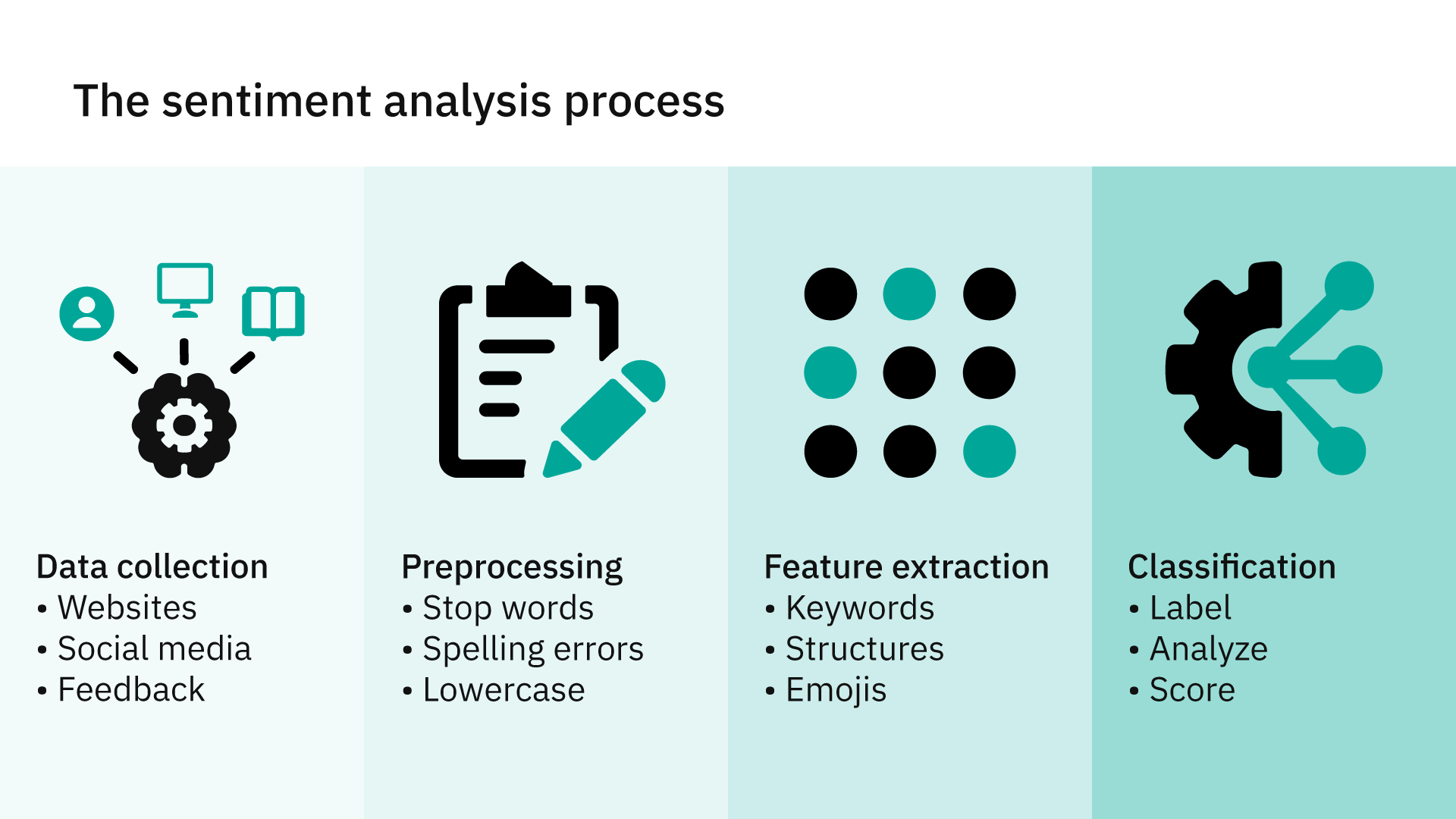
As the name suggests, it focuses on analyzing written text from various sources like social media posts, online reviews, customer feedback surveys, news articles, and more. It follows a systematic process:
- Data collection: The first step is to gather the relevant text data you want to analyze. This could involve scraping data from websites, using APIs to access social media feeds, or collecting feedback directly from customers.
- Preprocessing: Raw text data is often messy and needs to be cleaned up before analysis. This step involves tasks like removing stop words (common words like "the" or "and"), correcting spelling errors, and converting text to lowercase.
- Feature extraction: This is where NLP techniques come into play. The text is broken down into smaller chunks (words, phrases, or n-grams), and relevant features are identified. These features could be specific keywords, grammatical structures, or even emojis.
- Sentiment classification: This is the heart of sentiment analysis. AI algorithms, trained on vast amounts of labeled data, analyze the extracted features and assign a sentiment score to each piece of text. This score could be a simple positive/negative label or a more nuanced numerical value indicating the degree of positivity or negativity.
Essentially, once the tool has processed the data, each word, phrase or n-gram will be scored depending on its sentiment and an overall score will be calculated. Whilst initially many systems used rule-based models, these are becoming increasingly obsolete, and now the prevailing models will use NLP algorithms such as Naïve Bayes, Logistic Regression, Support Vector Machines, or Neural Networks. More on that below.
In the world of BPOs and outsourcing partners, companies can use sentiment analysis to determine the best ways to structure information, improve customer journeys, and continuously train agents and support teams. This kind of data is invaluable in constantly elevating the quality of offered CX.
The power of sentiment analysis AI models.
Various AI models are employed in sentiment analysis, each with its own strengths and weaknesses.
- Rule-based models: These models rely on pre-defined rules (e.g., keywords associated with positive or negative emotions) to determine sentiment. While simple to implement, they struggle with sarcasm, irony, and complex language.
- Machine Learning models: These models learn from labeled examples of text and their corresponding sentiments. They can handle more nuanced language, but require a large amount of training data.
- Deep Learning models: These models, particularly neural networks, can learn complex patterns in language and are particularly effective in capturing context and meaning. They have shown great promise in achieving higher accuracy in sentiment analysis.
The rise of AI has significantly improved the accuracy and speed of sentiment analysis. With advancements in natural language understanding, AI models are becoming better at detecting subtle cues, understanding context, and handling different language variations.
What is also crucial is the capacity to scale these models and use them on ever-growing compilations of data, coming from various channels. With the rise of omnichannel services and brands becoming accessible via every channel imaginable, sentiment analysis AI tools must be compatible with various forms of data and not deterred by the volumes of such information.
Speech sentiment analysis: beyond words.
While text sentiment analysis is more common, speech sentiment analysis is gaining traction, especially with the rise of voice assistants and audio content. Speech sentiment analysis extends the capabilities of sentiment analysis beyond the written word, focusing on the emotional nuances embedded within spoken language. By analyzing acoustic features like tone of voice, pitch, volume, and rhythm, AI-powered tools can decipher emotions conveyed through speech. This has opened up a new realm of applications, particularly in areas where voice interactions are prevalent.
Sentiment analysis AI plays a crucial role in analyzing audio data, converting speech to text, and then applying similar techniques as in text analysis to determine sentiment. This has applications in call center analytics, voice-based surveys, and even analyzing podcasts and interviews to understand audience reactions.
Call centers, for example, leverage speech sentiment analysis to evaluate customer satisfaction during calls, allowing for real-time interventions or follow-ups when negative sentiment is detected. Similarly, market researchers use it to gauge consumer reactions to new products or advertising campaigns through voice surveys. Virtual assistants and voice-controlled devices can also benefit from speech sentiment analysis to provide more empathetic and personalized responses.
However, this technology is not without its limitations. Accents, dialects, and background noise can pose challenges for accurate analysis, and the interpretation of emotions solely through acoustic features can be less precise than analyzing the actual words spoken in text format. Cultural nuances also play a part here, with linguistic idiosyncrasies providing potentially murky waters for interpretation of intention and tone. The dictionary definition of a word or phrase may be one thing, however commonly accepted and unregulated social norms may convey a different meaning.
While speech sentiment analysis faces these challenges, the advancement of AI, particularly in the form of Large Language Models (LLMs), offers promising solutions. LLMs are trained on massive datasets of text and code, enabling them to understand and generate human-like language with impressive accuracy.
In the context of speech sentiment analysis, LLMs can be fine-tuned on diverse datasets representing various accents, dialects, and cultural expressions. This allows them to learn the subtle differences in pronunciation, vocabulary, and intonation that can influence the interpretation of emotions in speech. By incorporating this knowledge, AI-powered speech sentiment analysis tools can become more robust and accurate, even when dealing with non-standard forms of speech. Additionally, LLMs can be used to generate synthetic speech data with different accents and dialects, further enriching the training data for sentiment analysis models and improving their ability to understand diverse forms of spoken language.
Interested in sentiment analysis AI?
Talk to usSentiment analysis applications.
Sentiment analysis has far-reaching applications across various industries, revolutionizing how businesses interact with their customers, understand market trends, and manage their reputation.
Customer experience.
Sentiment analysis has become an indispensable tool for understanding customer experience. By analyzing social media comments, online reviews, and customer feedback surveys, businesses can gain valuable insights into how their customers perceive their brand, products, or services.
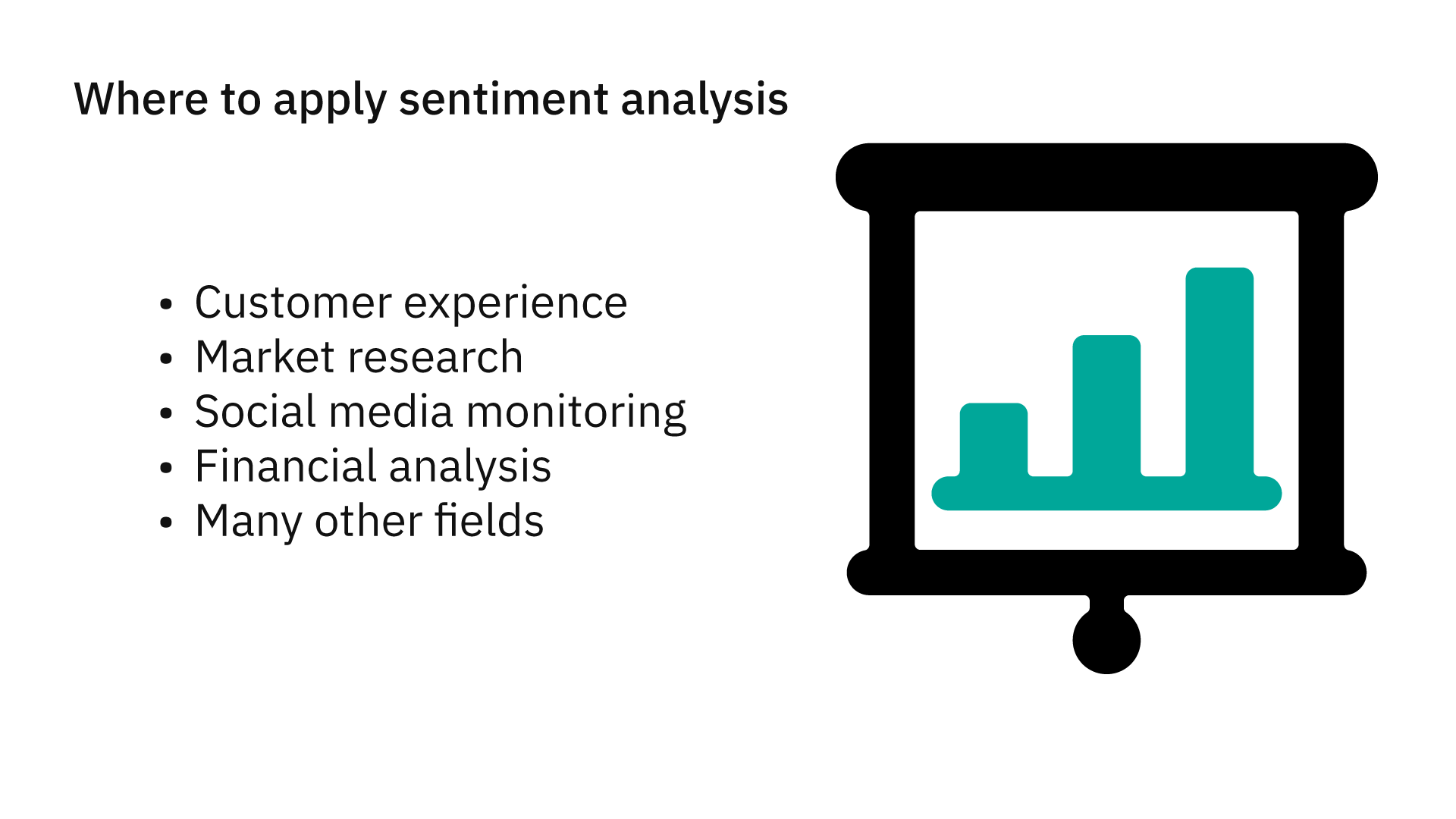
This allows companies to identify pain points, areas for improvement, and emerging trends. For example, a sudden surge in negative sentiment about a particular product feature could prompt a company to investigate and address the issue before it escalates into a crisis. Positive sentiment towards a service or product can trigger additional promotion to capitalize on the ‘hype’ while consumers are interested.
With this data, companies can also better train and upskill their own support teams and employees providing customer care services. With diverse datasets and AI applications, this can be made as accurate to local nuances as possible, whilst scaling business and retaining lower operating costs.
Market research.
Sentiment analysis helps businesses gain a deeper understanding of their target market by analyzing opinions and attitudes about products, competitors, or industry trends. By monitoring social media conversations and online forums, companies can identify emerging trends, customer preferences, and unmet needs.
This information is invaluable for product development, marketing campaigns, and overall business strategy. Sentiment analysis can also be used to track brand reputation and measure the effectiveness of marketing campaigns. As mentioned in the Pepsi ad example at the beginning of this blog, it can even save a brand from greater negative repercussions following an unsuccessful or tone-deaf marketing campaign.
Many companies use sentiment monitoring and brand mentioning tools to keep an eye on how their brand is being perceived, as well as look for gaps in the market that could be filled by new services or products. Sometimes it may even feel as though a brand has magically predicted a hot trend or topic, when in reality, it’s the culmination of millions of data points that have been meticulously analyzed to produce valuable insights. Again, this is a place where AI has excellent applications and can greatly increase the scalability of solutions.
Social media monitoring.
Social media has become a powerful platform for people to express their opinions and emotions. Sentiment analysis enables businesses to monitor social media conversations in real-time, tracking public sentiment about events, campaigns, or crises.
This can help companies respond quickly to negative feedback, identify potential PR risks, and gauge the overall public perception of their brand. For example, during a product launch, sentiment analysis can help assess public reaction and identify any potential issues early on. It can also help organizations prepare prior to launching new products with contingency plans, and monitor public response in realtime to allow for more appropriate reactions.
The world of content moderation, which has become hugely important since the advent of social media and gaming platforms in particular, is making more and more use of sentiment analysis AI tools in recent years. The highly sensitive and attuned nature of these tools allows for quick identification and response to negative sentiments, which in turn could be potentially harmful or against usage guidelines.
Financial analysis.
Sentiment analysis is also used in the financial sector to gauge investor sentiment by analyzing news articles, social media posts, and financial reports. This can provide valuable insights into market trends, predict stock price movements, and inform investment decisions.
For example, a sudden increase in negative sentiment about a particular company in financial news could indicate a potential downturn in its stock price. Investors can use this information to make informed decisions about their portfolio. Many modern day digital banks and online investment firms offer such services as part of their core products, providing a sense of security to users.
Beyond business: sentiment analysis in other fields.
The applications of sentiment analysis extend beyond the business world. In healthcare, it can be used to analyze patient feedback and improve the quality of care. In politics, it can be used to gauge public opinion on various issues and inform policy decisions. In education, it can be used to assess student engagement and identify areas where teaching methods can be improved.
Essentially, knowing how people are feeling and attempting to understand why they feel that way is no longer just a highly prized skill for top performing employees. It’s becoming vital to maintaining a positive brand reputation and increasing revenue and loyalty.
A closer look at text sentiment analysis.
While sentiment analysis can be applied to both text and speech, text data remains the most common form of data for analysis due to its abundance and accessibility. Text sentiment analysis offers various types of analysis:
- Fine-grained analysis.
While basic sentiment analysis classifies text as positive, negative, or neutral, fine-grained analysis delves deeper into the nuances of emotions and opinions. It aims to identify specific emotions like joy, anger, sadness, or fear, providing a more granular understanding of the sentiment expressed. Moreover, fine-grained analysis can pinpoint the target or aspect of the sentiment, determining what exactly the person is feeling positive or negative about.
For example, in a product review, fine-grained analysis can distinguish between positive sentiment towards the product's design and negative sentiment towards its battery life (e.g., "The battery life on this phone is amazing!" – the positive sentiment is specifically about the battery life).
This level of detail is invaluable for businesses as it allows them to identify specific areas for improvement and tailor their responses accordingly. By understanding not just whether a customer is happy or unhappy, but also why they feel that way, companies can make more informed decisions about product development, customer service, and marketing strategies.
- Intent Analysis.
Intent analysis, another facet of text sentiment analysis, focuses on deciphering the underlying goal or purpose behind a piece of text. It goes beyond simply understanding the emotions expressed and seeks to determine what the writer or speaker intends to achieve with their words. This involves categorizing text into different intents, such as requests, questions, complaints, or compliments.
Intent analysis is particularly valuable for applications like chatbots and virtual assistants, where understanding user intent is crucial for providing accurate and helpful responses. By identifying the intent behind a user's message, these AI-powered systems can route inquiries to the appropriate department, provide relevant information, or take specific actions.
For instance, if a customer asks, "How do I reset my password?" intent analysis would recognize this as a request for help and direct the user to the password reset instructions. This not only improves the efficiency of customer service but also enhances the overall user experience by providing more personalized and relevant interactions.
Sentiment analysis AI in action.
Although still an evolving element of many businesses, there are some excellent examples of companies implementing sentiment analysis tools, powered by AI, to improve the services they offer and streamline operations.
Ford, for example, uses advanced AI-driven sentiment analysis to check feedback from customers and gain valuable insights into vehicle performance. With this, Ford can spot concern areas, implement vehicle enhancements, and deliver better and better driving experiences in real-time to its customers, while maintaining their lead in the automotive industry.
T-Mobile, on the other hand, uses advanced AI sentiment analysis tools to better understand consumer behavior and anticipate trends or needs for their products or services. This way, they can provide a more personalized and accurate experience, which not only delights the customer, but decreases cost and resources spent on each interaction.
Common challenges in sentiment analysis.
Sentiment analysis, despite its advancements, still faces several challenges that can affect the accuracy and reliability of results.
- Sarcasm and irony: Humans often use sarcasm and irony to express their true feelings, which can be difficult for AI models to detect. For example, a comment like "Great job on the new design. It's so ugly, I love it!" could be misinterpreted as positive sentiment.
- Contextual understanding: The meaning of words and phrases can vary depending on the context. For example, the word "sick" could mean "ill" or "cool," depending on the surrounding words. AI models need to be able to understand context to accurately interpret sentiment.
- Language variations: Slang, dialects, abbreviations, and cultural references can pose challenges for sentiment analysis. AI models trained on standard language may struggle to understand these variations, leading to inaccurate sentiment scores.
- Data quality: The accuracy of sentiment analysis heavily relies on the quality of the input data. Inaccurate, incomplete, or biased data can lead to skewed results. For example, if a dataset is predominantly made up of positive reviews, the sentiment analysis model may overestimate the overall positive sentiment.
- Privacy concerns: The use of personal data for sentiment analysis raises ethical concerns about privacy and consent. Companies need to be transparent about how they collect and use data, and individuals should have the right to opt out if they choose.
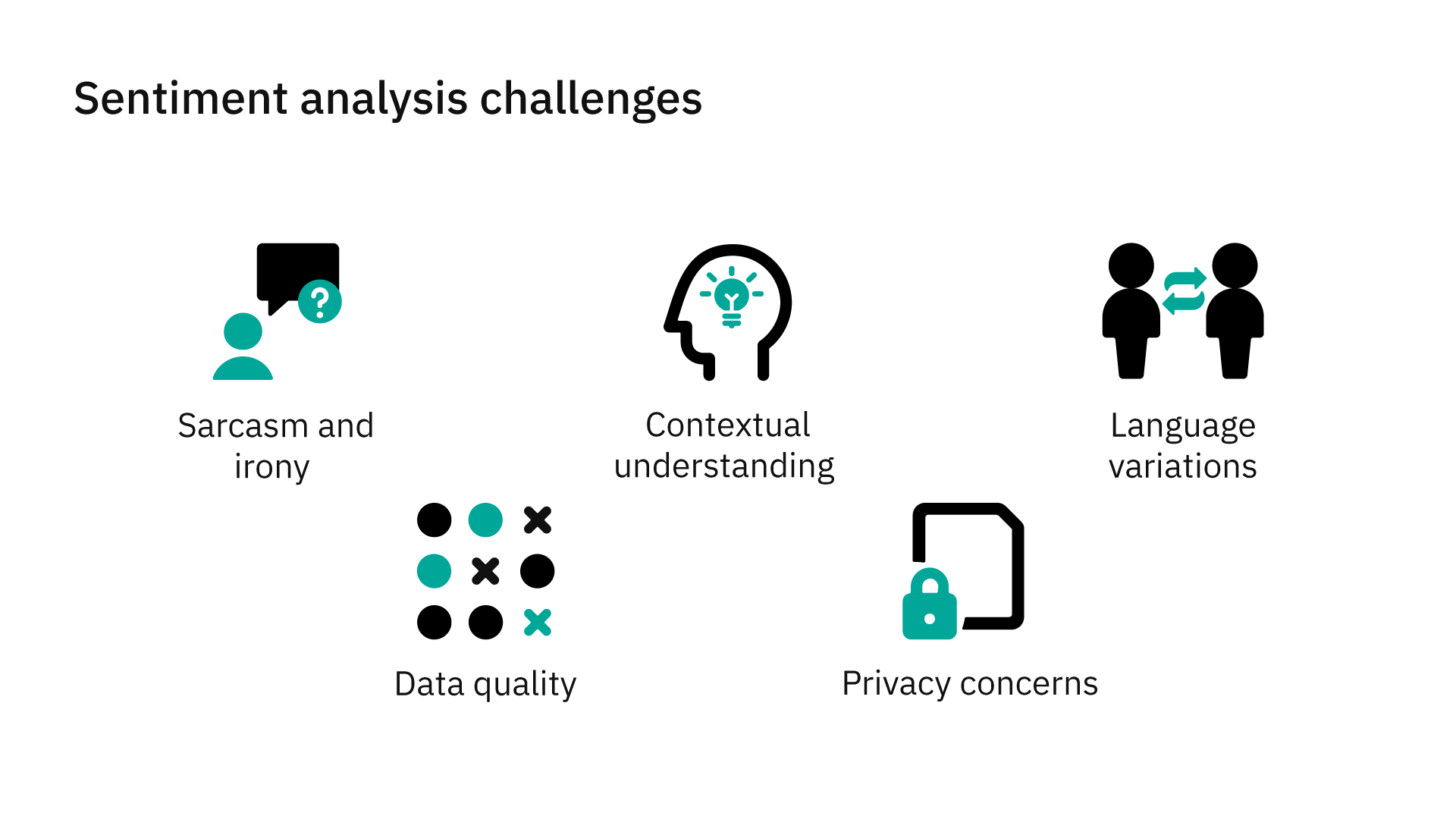
As this technology continues to evolve, addressing these challenges will be crucial to ensure its effectiveness and responsible application in understanding and responding to human emotions and opinions. AI can be a powerful tool in aiding businesses with each of these issues, however significant human oversight is still needed to ensure the highest quality of performance.
The role of AI in the future.
Advancements in AI are continuously pushing the boundaries of sentiment analysis, addressing these challenges and improving accuracy.
- Contextual AI models: New AI models are being developed that take into account the context of a statement, allowing them to better understand sarcasm, irony, and nuanced language. These models use techniques like attention mechanisms and transformers to weigh the importance of different words and phrases in a sentence.
- Transfer learning: This involves leveraging pre-trained models on large datasets and fine-tuning them for specific sentiment analysis tasks. This can help overcome the challenges of language variations and limited data.
- Human-in-the-loop validation: AI is not perfect, and human intervention is often necessary to validate and correct the results of sentiment analysis. This involves having human experts review and annotate data, providing feedback to AI models, and ensuring that the results align with real-world understanding.
- Hybrid models: Combining rule-based approaches with machine learning or deep learning can leverage the strengths of both. Rule-based systems can handle simple cases and provide interpretable results, while machine learning and deep learning can tackle complex language and nuances.
- Ethical considerations: As AI becomes more sophisticated, ethical considerations become increasingly important. Companies need to be transparent about how they use sentiment analysis, ensure that data is collected and processed responsibly, and avoid perpetuating biases in their algorithms.
The future of sentiment analysis.
The future of sentiment analysis is brimming with possibilities, as AI continues to evolve and push the boundaries of what's possible. No long simply a way to scale data volumes or prepare insights from a battery of information, AI is leading the way in actual interpretation, categorization, and even emotional intelligence.
There’s no telling where the limits are for sentiment analysis AI at this moment, but industry experts are excited about these advancements in particular:
- More nuanced understanding of emotions: AI models will become even better at understanding subtle nuances of emotions, going beyond simple positive/negative labels. This will allow for more accurate and detailed sentiment analysis, enabling businesses to gain deeper insights into customer opinions and attitudes.
- Real-time analysis and decision-making: Sentiment analysis will become more integrated into real-time decision-making processes. Businesses will be able to instantly analyze customer feedback, social media mentions, and other data sources to identify trends, respond to issues quickly, and make data-driven decisions on the fly.
- Multilingual sentiment analysis: As businesses expand globally, the need for multilingual sentiment analysis will grow. AI models will be able to analyze text and speech in multiple languages, enabling companies to understand customer sentiment across different cultures and regions.
- Emotionally intelligent AI: Sentiment analysis will become a core component of emotionally intelligent AI systems, allowing machines to understand and respond to human emotions more effectively. This could lead to more personalized customer service, more engaging chatbots, and even AI-powered therapists.
A note about AI and emotional intelligence.
The emergence of emotionally intelligent AI is poised to revolutionize sentiment analysis, unlocking new levels of understanding and nuance in deciphering human emotions. By incorporating elements of emotional intelligence, such as empathy, contextual awareness, and the ability to recognize subtle cues, AI systems can transcend the limitations of traditional sentiment analysis, which often struggles with sarcasm, irony, and complex emotions.
Emotionally intelligent AI can detect not only the polarity of sentiment (positive, negative, or neutral) but also the specific emotions conveyed, such as joy, sadness, anger, or fear. This deeper understanding can help businesses gain more actionable insights from customer feedback, social media conversations, and other text data.
For example, a company could identify customers who are not just dissatisfied but also angry, allowing them to prioritize and address those issues with greater urgency. Moreover, emotionally intelligent AI can personalize interactions based on detected emotions, leading to more empathetic customer service and enhanced user experiences.
There are, of course, ethical implications and concerns over data privacy, authenticity, and the potential dehumanization of certain traditionally intimate and very human processes. However, there are strong arguments to be made for the increased availability of certain services, lack of bias (provided tools are trained on diverse datasets), and reduced cost of such tools.
Sustainable sentiment.
Sentiment analysis is increasingly being applied to critical corporate social governance (CSG) topics, providing valuable insights into public perception, stakeholder engagement, and the effectiveness of initiatives. In the realm of sustainability, sentiment analysis can be used to monitor public opinion on environmental issues, track reactions to corporate sustainability reports, and assess the effectiveness of sustainability campaigns. By analyzing social media conversations, news articles, and other online discourse, companies can gauge public sentiment towards their sustainability efforts, identify areas for improvement, and tailor their communication strategies to resonate with stakeholders.
Similarly, sentiment analysis can be a powerful tool for understanding perceptions of diversity, equity, and inclusion (DEI) initiatives. By analyzing employee feedback, social media discussions, and news coverage, companies can gain insights into the effectiveness of their DEI programs, identify potential biases or blind spots, and measure the impact of their efforts on employee morale and engagement.
This data-driven approach can help companies create a more inclusive and equitable workplace, foster diversity, and enhance their reputation as responsible corporate citizens. Additionally, sentiment analysis can be applied to other CSG topics such as corporate governance, ethical practices, and social impact initiatives, providing valuable insights into stakeholder sentiment and guiding decision-making processes.
Effectiveness through diversity.
The effectiveness of sentiment analysis AI hinges on the quality and diversity of the data it's trained on. Training models on diverse datasets that encompass various demographics, dialects, writing styles, and cultural references is paramount to ensure accurate and unbiased results. When AI models are exposed to a broad range of language patterns and expressions, they become better equipped to understand and interpret sentiment across different contexts and demographics.
Neglecting data diversity can lead to biased algorithms that perform poorly on certain groups or fail to capture nuances in language specific to certain cultures or communities. For instance, a sentiment analysis model trained primarily on formal language might struggle to interpret slang or informal expressions commonly used in social media. Similarly, a model trained on data from a specific region might misinterpret sentiments expressed in other dialects or languages. By training on diverse datasets, AI models can avoid such biases and deliver more reliable and inclusive sentiment analysis results, catering to a wider range of users and applications.
In conclusion.
Sentiment analysis is not just a buzzword; it's a powerful tool that can unlock valuable insights from the vast amount of text and speech data generated every day. By understanding customer sentiment, businesses can improve their products and services, enhance customer experience, and make informed decisions that drive growth. While this tool is still evolving and faces challenges, advancements in AI are continuously pushing the boundaries of what's possible. By embracing this technology and leveraging its capabilities, businesses can gain a competitive edge in today's data-driven landscape. If you're looking to harness the power of sentiment analysis for your business, there are various tools and solutions available in the market. Whether you choose to build your own model or use a third-party service, investing in sentiment analysis is an investment in understanding your customers, your market, and your brand's reputation.