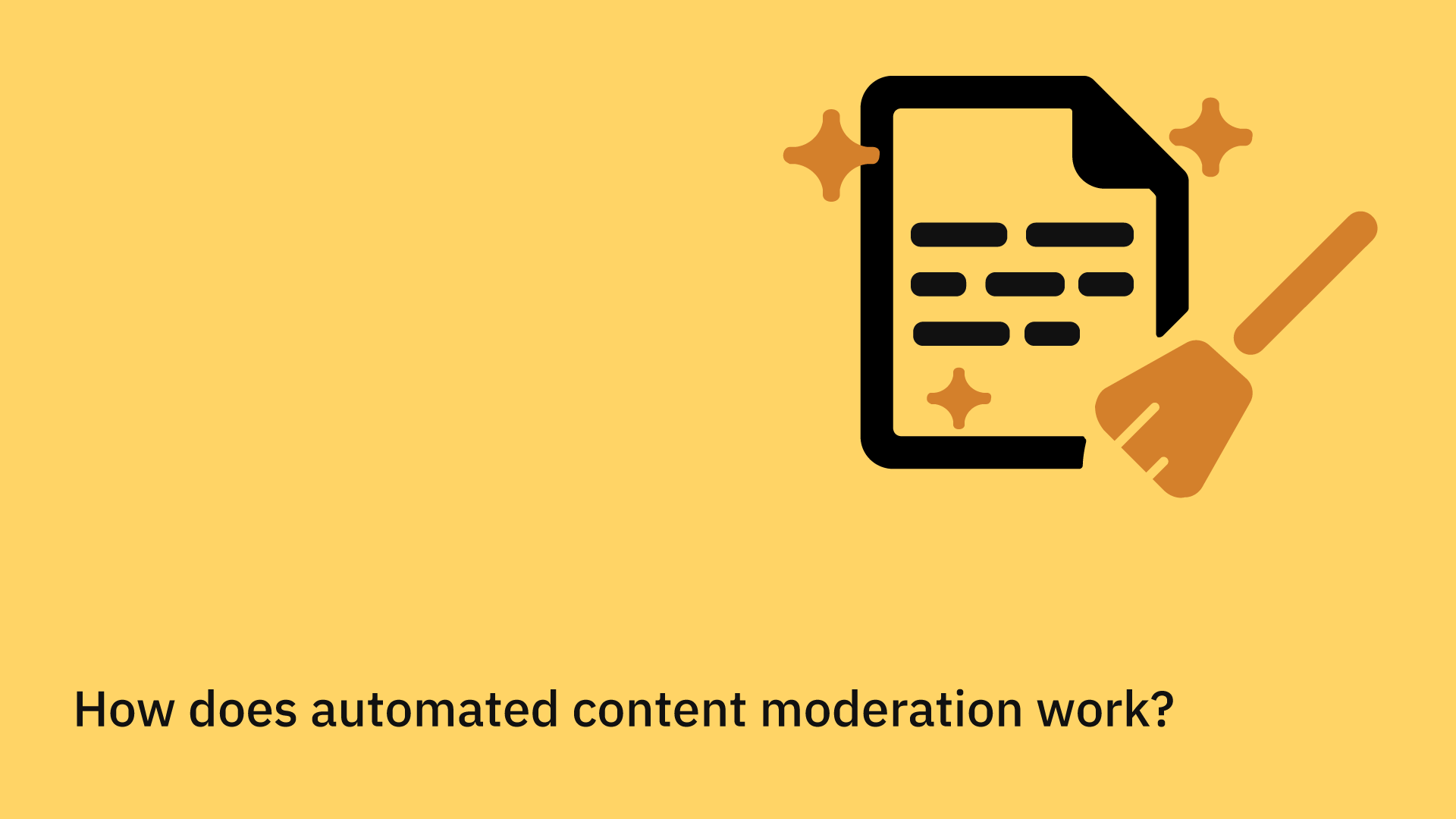
How automated content moderation works.
Updated on January 15, 2025
Content moderation has been a necessity since the first instances of user-generated material. However, the sheer volume and velocity of content creation make manual moderation nearly impossible. Enter automated content moderation, a game-changing technology that leverages artificial intelligence (AI) and algorithms to streamline the process.
With many social media platforms and sites now reaching deep into the billions of users, effective and accurate automation of this process has companies developing ever-more complex tools and systems. Meta has reported that it no longer relies on user reports, but automation tools to identify 97% of content that violates hate speech policies.
This comprehensive guide will delve into the intricacies of automated content moderation, exploring how it works, its evolution, different types, benefits, limitations, and the future it holds.
What is automated content moderation?
At its core, automated content moderation involves using AI and machine learning algorithms to automatically identify and filter out inappropriate or undesirable content from online platforms. This includes content that may be violent, hateful, sexually explicit, or spam-like. The goals are clear: protect users from unwanted content, maintain a safe and welcoming online environment, and ensure compliance with legal and regulatory requirements.
Automated content moderation is less and less rare, finding applications across various platforms like social networks, e-commerce sites, news outlets, and gaming communities. Its importance is only set to grow as the volume of online content continues to surge.
How does it work?
Every organization defines a process that works best for its purposes and user base. Firstly, you have to determine when the moderation will take place:
- Pre-moderation: the tools and algorithms in use screen all content before it gets published.
- Post-moderation: user-generated content is screened quickly after going live. This is by far the most popular method as it allows users to publish content instantly, yet still maintains a rapid response time.
- Reactive moderation: the platform relies on users reporting inappropriate posts after they’ve been published.
Of course, each of these routes comes with its own benefits and challenges. Pre-moderation is safer and can result in fewer materials that violate platform guidelines being published, however might seem inhibitive to users. Post-moderation risks inappropriate content being live for a limited time - during which it can still be seen by many users - yet allows for a less restrictive user experience. And reactive moderation relies on the community to react to content and make their own decisions, which informs the platform of what users believe to be harmful - but can be biased and unreliable.
When it comes to the process of filtering - although it can differ slightly depending on the industry, platform, or provider, the workflow of automated content moderation typically follows these steps:
- Content upload: A user uploads content, whether it's a comment, post, image, or video, to an online platform.
- Automated screening: AI algorithms immediately scan the content, analyzing it for specific keywords, phrases, patterns, and even visual cues that might indicate its inappropriateness.
- Filtering or flagging: If the content is deemed to violate the platform's guidelines, it can be automatically removed, hidden from public view, or flagged for further review.
- Human review (optional): In some cases, content flagged by AI undergoes additional scrutiny by human moderators to ensure accuracy and avoid mistakenly removing harmless content.
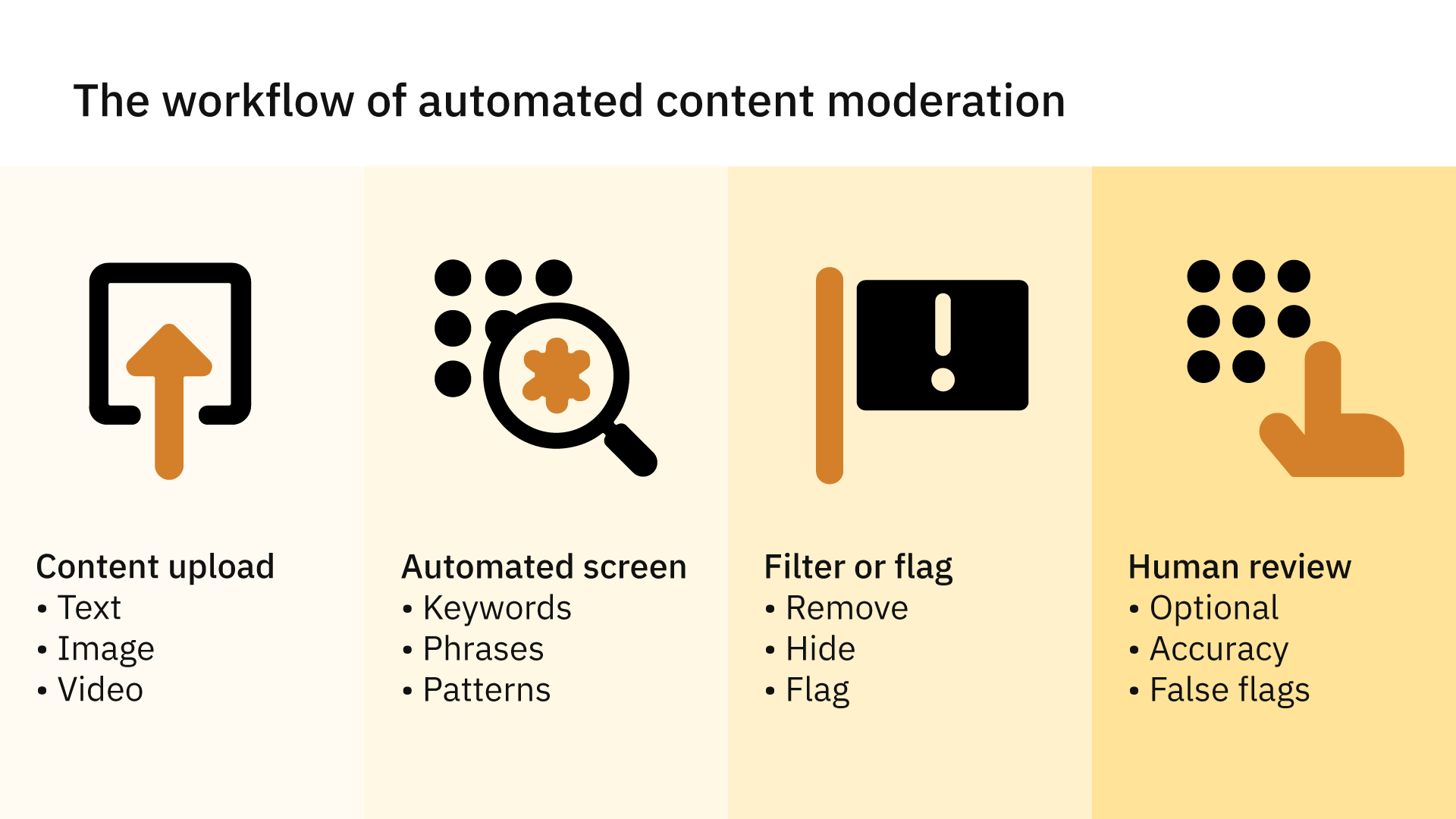
The algorithms used in automated content moderation often rely on natural language processing (NLP) to understand the meaning and context of text. Image and video moderation might use computer vision to identify inappropriate visual content.
This is where Large Language Models (LLMs), advanced AI software, and open sourced platforms such as OpenAI’s ChatGPT have been changing the game. Going from simpler automation to intricate identification using models trained on specific datasets can allow platforms to increase effectiveness while decreasing costs of maintaining large teams of content moderators. Instead, AI can do the heavy lifting, and the human touch is there to guide and confirm.
From automated to AI.
The early days of automated content moderation were characterized by simple rule-based systems that searched for specific keywords or phrases. These systems were limited in their ability to understand context and nuance, often resulting in inaccurate filtering.
Advancements in machine learning and AI have revolutionized the field. AI-powered moderation systems can now learn and adapt, improving their accuracy over time. They can understand more complex language patterns, recognize subtle cues, and make more nuanced judgments about content. Situations that previously might have resulted in “glitches” or “hallucinations” on the part of AI systems are becoming less common, as these tools are trained to recognize nuance and understand cultural idiosyncrasies.
Through the use of AI-powered knowledge bases, such as the ones used by Transcom, it’s possible to tailor the algorithm to any specific industry or dataset, resulting in a more effective content moderation operation at a lower cost.
The types of AI content moderation.
- Text-based moderation: This is the most common type, focusing on analyzing written content like comments, posts, and reviews. It can detect hate speech, cyberbullying, spam, and other forms of inappropriate text. Algorithms look for specific words that they have been trained to pick up on, or even use text sentiment analysis to determine the intent of written content.
- Image-based moderation: This type analyzes images and videos for inappropriate content, such as violence, nudity, or hate symbols. Computer vision is used for these purposes, through object detection mechanisms that can recognise not only individual elements, but also how they fit into the scene and their significance.
- Video moderation: The most complex of all types of moderation, video requires every frame and image in the video to be analyzed for potentially harmful content. It may also be necessary to check the audio of a video clip, which adds a layer of complexity to the process.
Across all of these types of AI content moderation, techniques such as digital hashing are used to separate, group, and identify bits of information for analysis. Essentially, hashes act like fingerprints for text, images, or video, and platforms can use these hashes to quickly check content even after it has already been edited. Even more advanced methods, called perceptual hashing, allow for the tagging of signature data that has “survived” alteration, and flags it to the system for analysis.
Other factors like the accuracy of metadata also influence the accuracy of automated content moderation tools, as does the level of training an AI-powered tool receives before it’s put to work.
The benefits of automated content moderation.
Automated content moderation offers several compelling benefits:
- Efficiency: It can process vast amounts of content in a fraction of the time it would take human moderators, ensuring swift action against undesirable content.
- Scalability: As platforms grow and user-generated content increases, automated systems can easily scale to meet the demand.
- Consistency: AI algorithms apply the same set of rules and criteria to all content, reducing the risk of human bias and ensuring fair treatment.
- Cost-effectiveness: While AI systems require initial investment, they are often more cost-effective in the long run compared to hiring and training large moderation teams.
- Protection of users: By quickly removing unwanted content, automated moderation helps protect users from exposure to offensive or dangerous material.
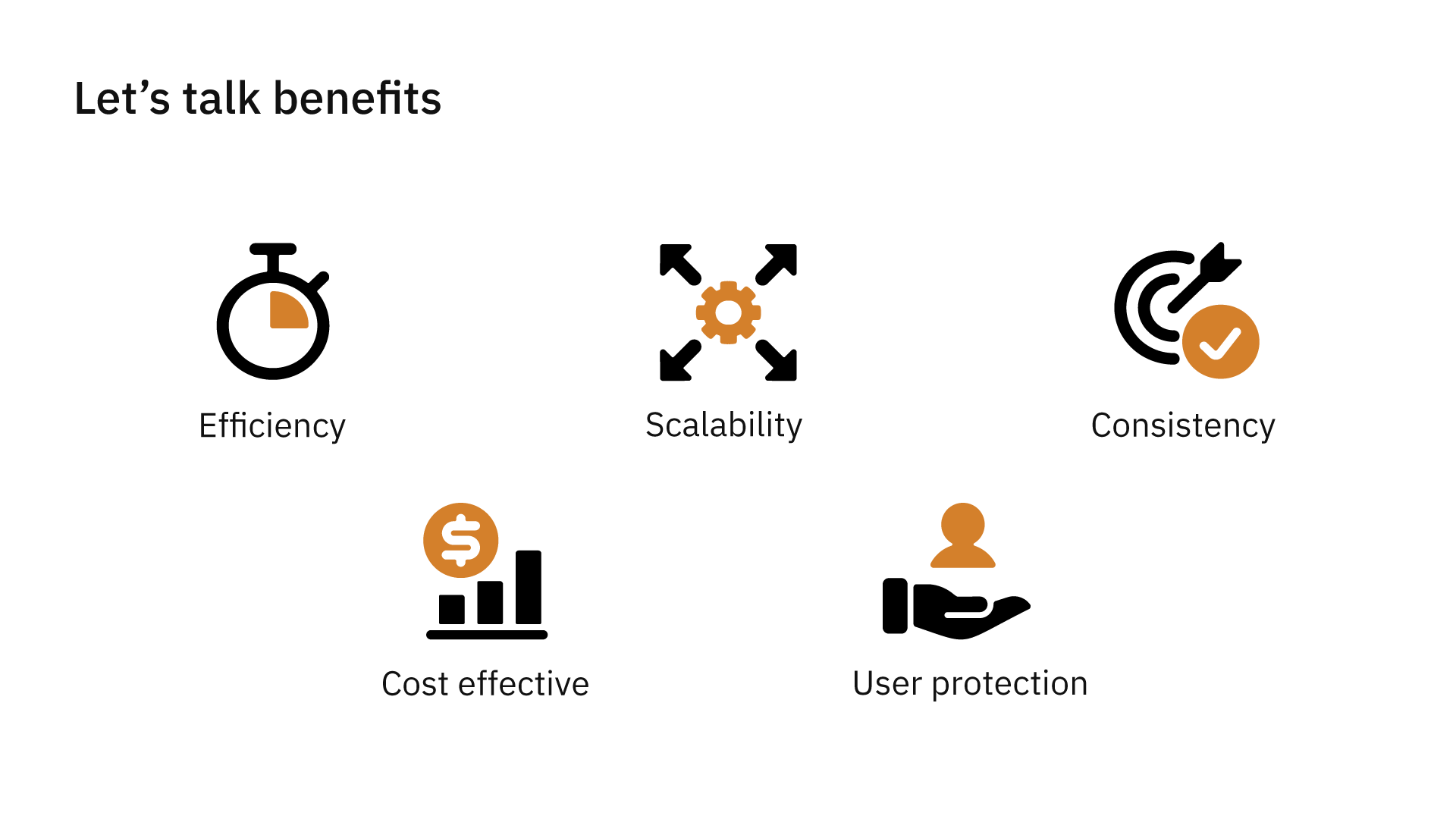
The limitations of automated content moderation.
Despite its advantages, automated content moderation is not without its limitations:
- Accuracy: Even the most sophisticated AI algorithms can make mistakes, leading to false positives (removing harmless content) or false negatives (missing unwanted content).
- Context: AI may struggle to understand the context of certain expressions, such as sarcasm or humor, leading to incorrect filtering decisions.
- Bias: If the training data used to develop the AI model is biased, the model itself can perpetuate those biases in its decision-making.
- Over-reliance: Relying solely on automated systems can lead to over-dependence and neglect the importance of human judgment in complex cases.
- Sustainability concerns: Running large scale AI computing operations is associated with increased carbon emissions and environmental risk.
Whilst these limitations are important to acknowledge, they can be mitigated with a strategy that is targeted, tailored, and thoughtful in its approach.
The future of automated content moderation.
The field of automated content moderation is constantly evolving. Ongoing research and development are focused on creating more sophisticated algorithms that can better understand context, detect subtle cues, and make more accurate decisions.
Ethical considerations and responsible AI development are crucial in this field. Ensuring transparency, fairness, and accountability in automated moderation systems will be paramount as they become even more integrated into our online experiences. Data privacy, the protection of consumer interests as well as business priorities, and ensuring a positive user experience in potentially “charged” environments will become increasingly challenging and vital to keep users coming back.
The future of content moderation likely lies in a hybrid model, where AI handles the bulk of the screening and filtering, while human moderators focus on complex cases and fine-tune the algorithms. This AI-led, human-governed approach may provide the ideal middle ground between the large scale capacity of AI models and the nuanced understanding we’ve come to expect from human content moderators.
In conclusion.
Automated content moderation is a powerful tool in the fight against unwanted online content. Its efficiency, scalability, and consistency make it an invaluable asset for platforms of all sizes. However, it's important to acknowledge and address its limitations, particularly in terms of accuracy, context, and bias. The future of automated content moderation is AI. With ongoing advancements promising even more effective and nuanced systems, it’s feasible that we’ll soon see even faster reaction times, less associated cost, and higher accuracy. By striking the right balance between AI and human expertise, we can create safer and more welcoming online communities for everyone.